About Us
- Home
- About US
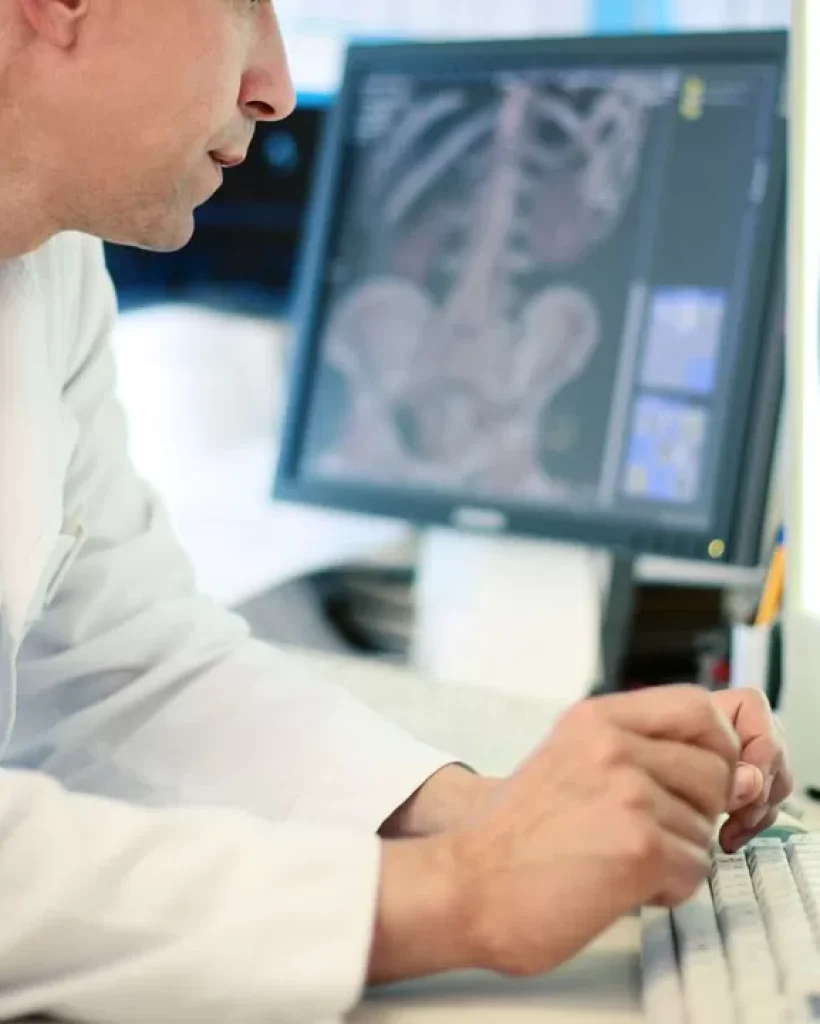
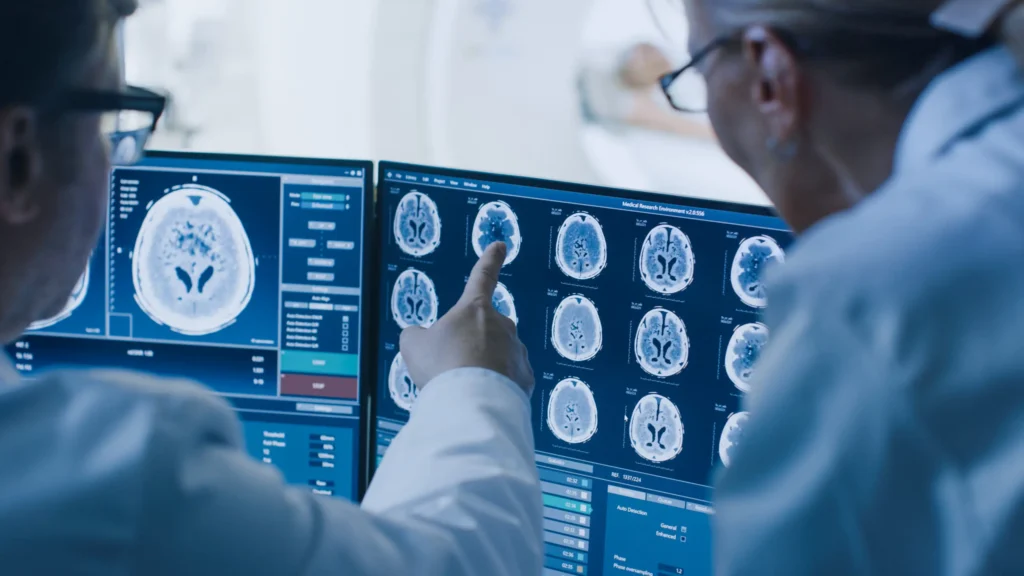
About Radexa
We Provide Essential Services For Your Health Care
Artificial intelligence (AI) and Tele-Radiology Services (TRS) offer the Up-To-Date Radiology solutions all over the world by enabling the achievement of remote image interpretation anywhere, anytime.
AI, particularly its subset machine learning, is radically improving radiology, strengthening image analysis, and mitigating diagnostic errors. AI algorithms process and interpret data, performing tasks that emulate or even surpass human cognitive capabilities.
Through our website, we can provide you with distinguished services. We can search for and provide you with a group of radiology doctors who are located in your vicinity, and we can enter into a partnership and employment between your radiology department/ center and local radiologists to provide the radiology department/center with high-efficiency radiology services.
We can coordinate to inform you of the latest artificial intelligence devices in the field of diagnostic radiology that benefit diagnosis, speed up the performance of services, and help doctors provide radiology services with high efficiency.
AI TOOLS IN RADIOLOGY
unlock a significant amount of potential for the AI radiology companies
One of the most rapidly growing startups at the intersections of technology, artificial intelligence (AI) and radiology. For example, Internet clouds scale its efforts to improve clinical radiologists’ workflows and mitigate burnout. The AI radiology companies have numerous offerings for diagnosis and reporting platforms, which have been developed as a means to augment clinician productivity. The AI radiology companies automatically generate report impressions once the raw findings have been dictated by the radiologist. The key differentiator of this technology is that the platforms continuously learn from each user and generate a personalized output that is in the stylistic tone and literary voice of each individual radiologist.
Many tools help unlock a significant amount of potential for the AI radiology companies by increasing the accuracy of reports, improving stylistic customizations and contributing to workflow optimization.
Artificial intelligence (AI) has recently made substantial strides in perception (the interpretation of sensory information), allowing machines to better represent and interpret complex data. This has led to major advances in applications ranging from web search and self-driving vehicles to natural language processing and computer vision, tasks that until a few years ago could be done only by humans.
Deep learning is a subset of machine learning that is based on a neural network structure loosely inspired by the human brain.
While most earlier AI methods have led to applications with subhuman performance, recent deep learning algorithms are able to match and even surpass humans in task-specific applications. This is owing to recent advances in AI research, the massive amounts of digital data now available to train algorithms and modern, powerful computational hardware.
Following the trend towards a human-level general AI, researchers predict that AI will automate many tasks, including translating languages, writing best-selling books and performing surgery, all within the coming decades.
Many tools help unlock a significant amount of potential for the AI radiology companies by increasing the accuracy of reports, improving stylistic customizations and contributing to workflow optimization.
Artificial intelligence (AI) has recently made substantial strides in perception (the interpretation of sensory information), allowing machines to better represent and interpret complex data. This has led to major advances in applications ranging from web search and self-driving vehicles to natural language processing and computer vision, tasks that until a few years ago could be done only by humans.
Deep learning is a subset of machine learning that is based on a neural network structure loosely inspired by the human brain.
While most earlier AI methods have led to applications with subhuman performance, recent deep learning algorithms are able to match and even surpass humans in task-specific applications. This is owing to recent advances in AI research, the massive amounts of digital data now available to train algorithms and modern, powerful computational hardware.
Following the trend towards a human-level general AI, researchers predict that AI will automate many tasks, including translating languages, writing best-selling books and performing surgery, all within the coming decades.
The drivers
The Surge of AI in Medical Imaging for Enhanced Efficiency and Precision
The primary driver behind the emergence of AI in medical imaging has been the desire for greater efficacy and efficiency in clinical care. Radiological imaging data continues to grow at a disproportionate rate when compared with the number of available trained readers, and the decline in imaging reimbursements has forced health-care providers to compensate by increasing productivity. These factors have contributed to a dramatic increase in radiologists’ workloads. Studies report that, in some cases, an average radiologist must interpret one image every 3-4 seconds in an 8-hour workday to meet workload demands. As radiology involves visual perception as well as decision making under uncertainty, errors are inevitable, especially under such constrained conditions.
A seamlessly integrated AI component within the imaging workflow would increase efficiency, reduce errors and achieve objectives with minimal manual input by providing trained radiologists with pre-screened images and identified features. Therefore, substantial efforts and policies are being put forward to facilitate technological advances related to AI in medical imaging. Almost all image-based radiology tasks are contingent upon the quantification and assessment of radiographic characteristics from images. These characteristics can be important for the clinical task at hand, that is, for the detection, characterization or monitoring of diseases. The application of logic and statistical pattern recognition to problems in medicine has been proposed since the early 1960s. As computers became more prevalent in the 1980s, the AI-powered automation of many clinical tasks has shifted radiology from a perceptual subjective craft to a quantitatively computable domain. The rate at which AI is evolving radiology is parallel to that in other application areas and is proportional to the rapid growth of data and computational power.
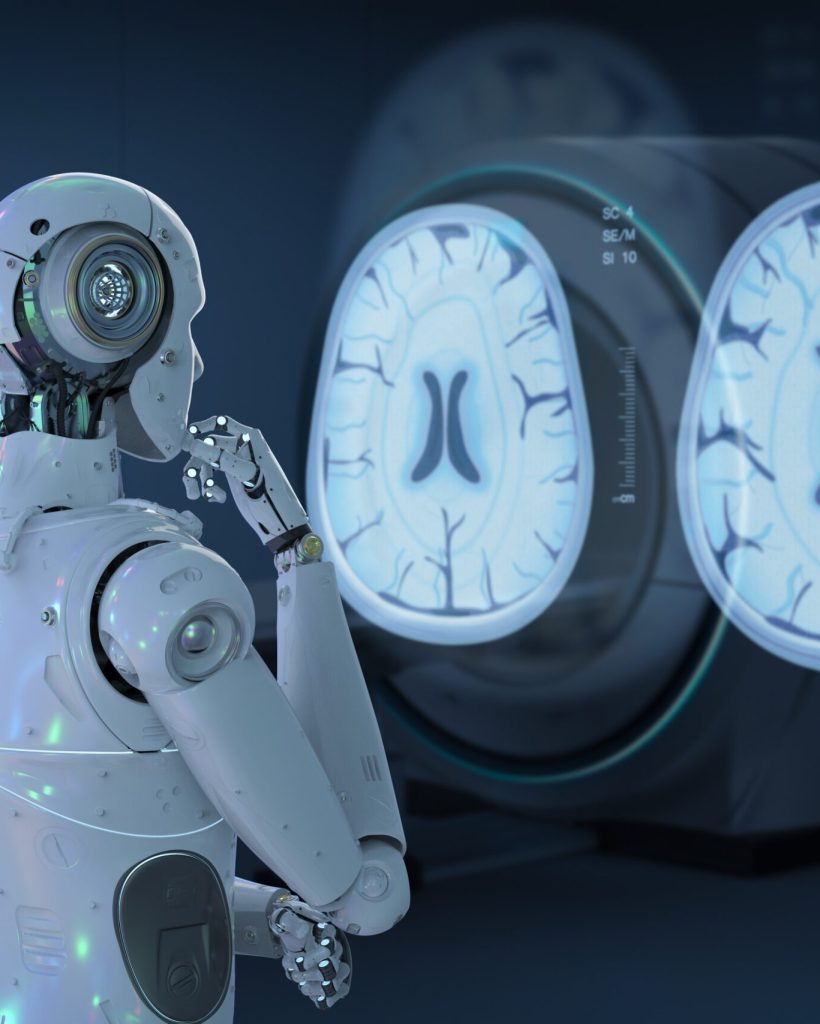
Two classes
There are two classes of AI methods that are in wide use today
The first uses handcrafted engineered features that are defined in terms of mathematical equations (such as tumor texture) and can thus be quantified using computer programs. These features are used as inputs to state-of-the-art machine learning models that are trained to classify patients in ways that can support clinical decision making. Although such features are perceived to be discriminative, they rely on expert definition and hence do not necessarily represent the most optimal feature quantification approach for the discrimination task at hand. Moreover, predefined features are often unable to adapt to variations in imaging modalities, such as computed tomography (CT), positron emission tomography (PET) and magnetic resonance imaging (MRI), and their associated signal-to-noise characteristics.
The second method, deep learning, has gained considerable attention in recent years. Deep learning algorithms can automatically learn feature representations from data without the need for prior definition by human experts. This data-driven approach allows for more abstract feature definitions, making it more informative and generalizable. Deep learning can thus automatically quantify phenotypic characteristics of human tissues, promising substantial improvements in diagnosis and clinical care. Deep learning has the added benefit of reducing the need for manual preprocessing steps. For example, to extract predefined features, accurate segmentation of diseased tissues by experts is often needed. Because deep learning is data driven, with enough example data, it can automatically identify diseased tissues and hence avoid the need for expert-defined segmentations. Given its ability to learn complex data representations, deep learning is also often robust against undesired variation, such as the inter-reader variability, and can hence be applied to a large variety of clinical conditions and parameters. In many ways, deep learning can mirror what trained radiologists do, that is, identify image parameters but also weigh up the importance of these parameters on the basis of other factors to arrive at a clinical decision.
For more information:
Artificial intelligence in radiology, Ahmed Hosney et. al, 2018.
TELERADIOLOGY
What to be?
Teleradiology refers to the practice of a radiologist interpreting medical images while not physically present in the location where the images are generated. Hospitals, mobile imaging companies, urgent care facilities and even some private practices utilize teleradiology.
The main idea behind the use of teleradiology is that it is expensive to have a radiologist on-site. On the other hand, using a teleradiology service enables you to pay on a per exam basis, and sometimes the cost is low and the service is available for longer time. For facilities requiring 24/7 services, or those that have a small volume of radiology patients, it can provide a huge savings.
Patient care is improved by teleradiology by making it possible for radiologists to provide their services without needing to be in the same location as the patient. That is especially important whenever a sub-specialist like a musculoskeletal radiologist, pediatric radiologist, neuroradiologist or MRI radiologist is needed, given that usually these professionals work in large metropolitan areas during day hours. However, teleradiology makes it possible to have access to trained specialists on a 24/7 basis.
In order to define exactly what teleradiology is, a good place to start is defining the word radiology first. Radiology is a type of imaging technique that physicians use for taking images of the internal part of the body. This is usually done for either treatment or diagnosis. Ultrasounds, MRIs and x-rays are examples.
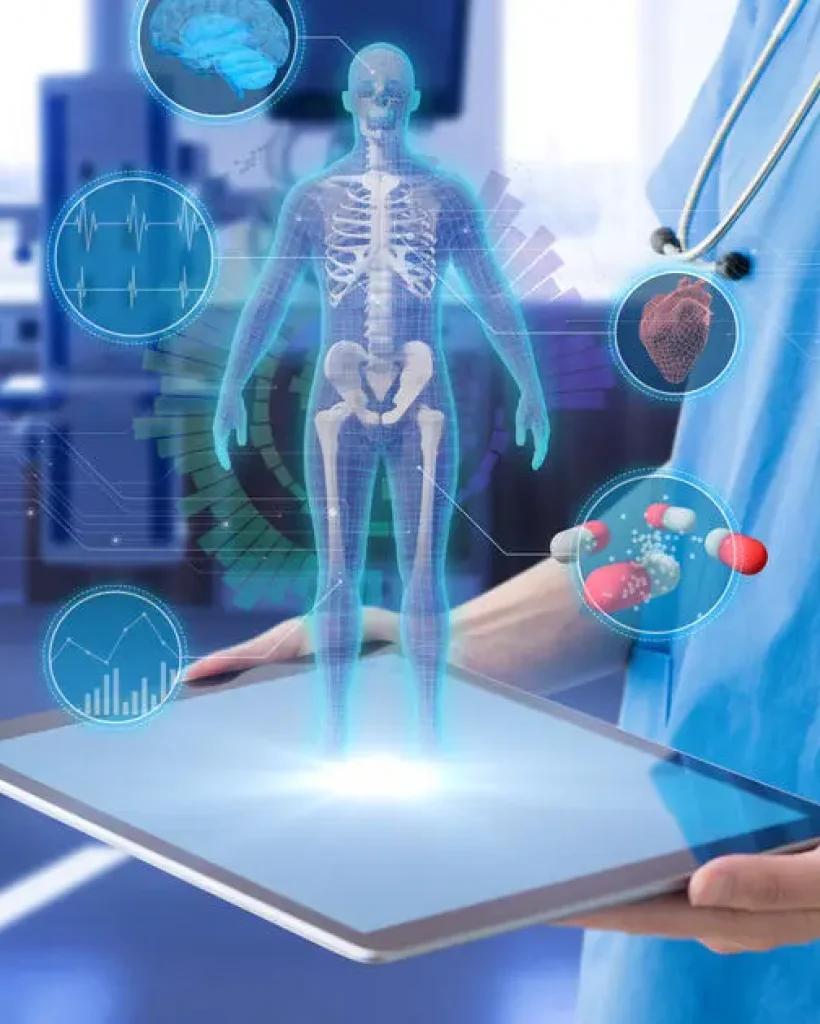
Drawbacks
However, like everything else, the practice can have some down sides to it. There is only so much a radiologist can do with images. Usually, the doctor will follow up on procedures. With teleradiology, however, the radiologist only receives images and there isn’t a patient to perform any additional tests on. That means the doctor will need to relay his information to the doctors that are on-site. That can lead to miscommunication and confusion.
Another obvious drawback to teleradiology is that it is dependent on technology. Teleradiology is impossible to do without technology. For example, if the internet is down at the hospital, teleradiology will not be possible to use. This could result in a delay in diagnosis and treatment.
Internet revolution
The Rapid Evolution and Crucial Impact of Teleradiology
Up until very recently, teleradiology was only used in emergencies. Of course, with the introduction of the internet, this practice started to spread very fast. It is as easy to send images as it is to send emails that have attachments. There are now computer programs that are dedicated specifically to the transmission of radiological images. These developments result in teleradiology turning into a significant medical practice, and it continues to grow in importance.
A doctor may want to use teleradiology in order to collaborate and consult with other doctors who are in other locations that he may not be able to without the technology. It helps with diagnosis, and frequently helps with getting a second opinion and assist with symptom control. Many times, it might be the first opinion since there might not be a radiologist available in the hospital.
One common scenario that often takes place is a patient coming into the emergency room. However, smaller hospitals may employ just one radiologist. Teleradiology makes it possible for the images that the ER teams takes to be examined by a radiologist located in a different location. This is particularly useful in rural areas.
What is really great about teleradiology is that a trained radiologist can read certain images and make life-saving discoveries. If an untrained eye views the images this isn’t always so. An ultrasound that shows a malignant growth might not mean anything to an untrained eye. However, it could indicate cancer to a trained radiologist. Teleradiology is the difference between a patient receiving the treatment the person needs, or leaving the hospital without getting a treatment plan.
History of Teleradiology
Transformative Timeline: The Evolution and Milestones of Teleradiology
Teleradiology has a history of almost a half a century at this point, and has been influential in forming the entire telemedicine industry.
Teleradiology during the early 1980s existed primarily as physical film copies that were mailed to the radiologist. Then he would record a report on a cassette tape. This would then be mailed to the original facility so that it could be transcribed into the form of a paper report. These studies’ turnaround time would take days and sometimes even weeks. For routine studies that would work, but that timeframe won’t cut it in emergency situations.
Although some modalities (X-ray machines, MRIs, CTs) produced films or digital images that could be scanned into a digital image, displaying the digital copies on machines made by other manufacturers was very difficult. The National Electrical Manufacturers Association and American College of Radiology in 1983 joined forces and created an open standard for medical images to be digitally stored. The ACR/NEMA 300 standard did have some limitations and problems that didn’t lead to manufacturers adopting it broadly.
A second version of this standard got released in 1988. It is usually called ACR/NEMA V2.0. With this version, the images were transmitted through using a dedicated 2 cable (the EIA-485). The standard was accepted more widely, with the standard being support by companies like 3M, Vortech (which is now Kodak), Siemens Medical Systems, Merge Technologies, General Electric Medical Systems and DeJarnette Research Systems.
The third version of this standard got released in 1993, and was called DICOM. Standard network support got added with this edition, which finally made teleradiology really possible.
Many tools help unlock a significant amount of potential for the AI radiology companies by increasing the accuracy of reports, improving stylistic customizations and contributing to workflow optimization.
Artificial intelligence (AI) has recently made substantial strides in perception (the interpretation of sensory information), allowing machines to better represent and interpret complex data. This has led to major advances in applications ranging from web search and self-driving vehicles to natural language processing and computer vision, tasks that until a few years ago could be done only by humans.
Deep learning is a subset of machine learning that is based on a neural network structure loosely inspired by the human brain.
While most earlier AI methods have led to applications with subhuman performance, recent deep learning algorithms are able to match and even surpass humans in task-specific applications. This is owing to recent advances in AI research, the massive amounts of digital data now available to train algorithms and modern, powerful computational hardware.
Following the trend towards a human-level general AI, researchers predict that AI will automate many tasks, including translating languages, writing best-selling books and performing surgery, all within the coming decades.
Many tools help unlock a significant amount of potential for the AI radiology companies by increasing the accuracy of reports, improving stylistic customizations and contributing to workflow optimization.
Artificial intelligence (AI) has recently made substantial strides in perception (the interpretation of sensory information), allowing machines to better represent and interpret complex data. This has led to major advances in applications ranging from web search and self-driving vehicles to natural language processing and computer vision, tasks that until a few years ago could be done only by humans.
Deep learning is a subset of machine learning that is based on a neural network structure loosely inspired by the human brain.
While most earlier AI methods have led to applications with subhuman performance, recent deep learning algorithms are able to match and even surpass humans in task-specific applications. This is owing to recent advances in AI research, the massive amounts of digital data now available to train algorithms and modern, powerful computational hardware.
Following the trend towards a human-level general AI, researchers predict that AI will automate many tasks, including translating languages, writing best-selling books and performing surgery, all within the coming decades.